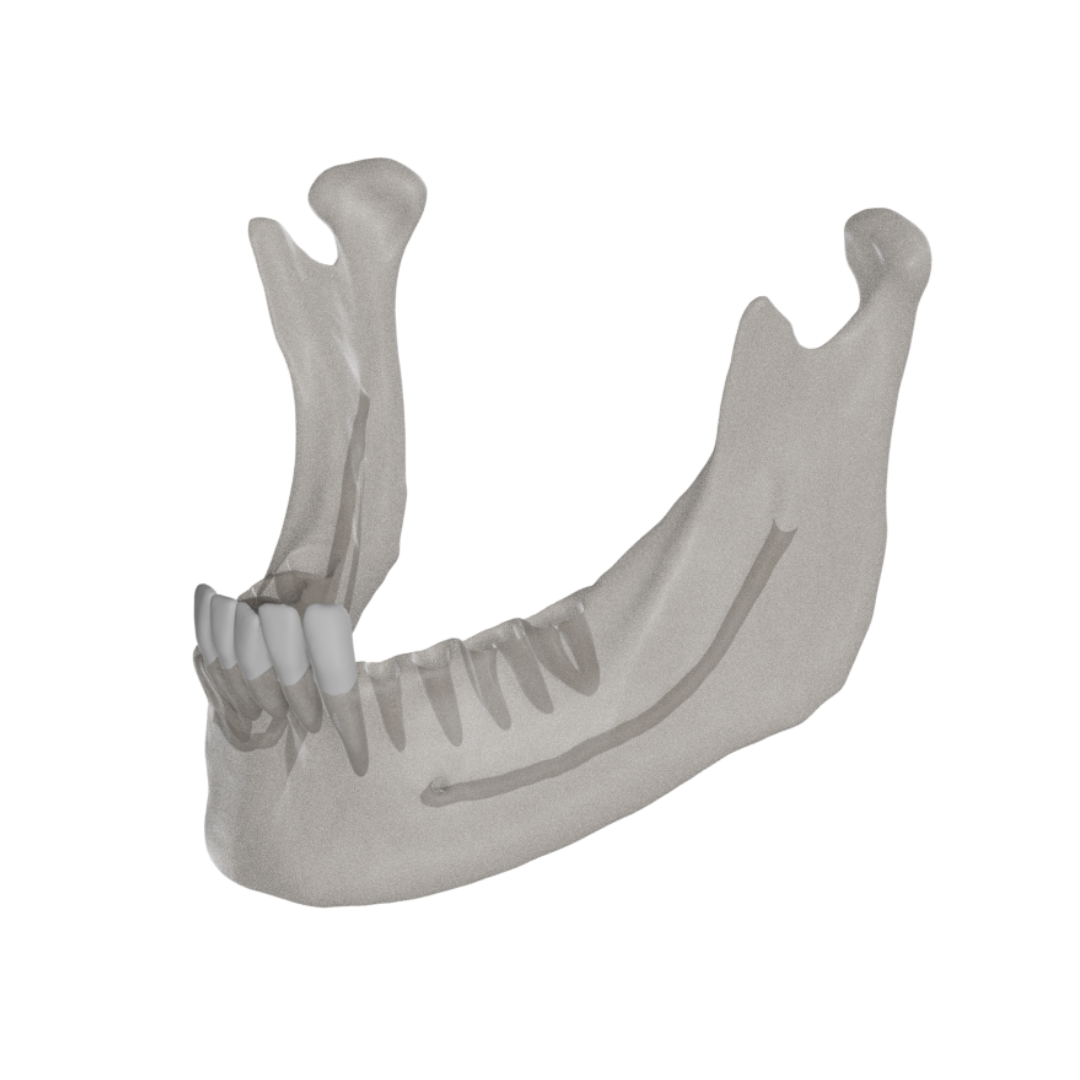
Link to paper
Automated detection and labelling of teeth and small edentulous regions on cone-beam computed tomography using convolutional neural networks
Maurício do Nascimento Gerhardt a b, Rocharles Cavalcante Fontenele a c, André Ferreira Leite a d, Pierre Lahoud a, Adriaan Van Gerven e, Holger Willems e, Andreas Smolders e, Thomas Beznik e, Reinhilde Jacobs a f
a OMFS IMPATH Research Group, Department of Imaging and Pathology, University of Leuven and Department of Oral & Maxillofacial Surgery, University Hospitals Leuven, KU Leuven, Kapucijnenvoer 33, Leuven 3000, Belgium
b School of Health Sciences, Faculty of Dentistry, Pontifical Catholic University of Rio Grande do Sul, Porto Alegre 90619-900, Brazil
c Department of Oral Diagnosis, Division of Oral Radiology, Piracicaba Dental School, University of Campinas, Piracicaba, Sao Paulo, Brazil
d Department of Dentistry, Faculty of Health Sciences, Campus Universitário Darcy Ribeiro, University of Brasília, Brasília 70910-900, Brazil
e Relu BV, Leuven, Belgium
f Department of Dental Medicine, Karolinska Institutet, Stockholm, Sweden
Abstract
Objective
To assess the accuracy of a novel Artificial Intelligence (AI)-driven tool for automated detection of teeth and small edentulous regions on Cone-Beam Computed Tomography (CBCT) images.
Materials and Methods
After AI training and testing with 175 CBCT scans (130 for training and 40 for testing), validation was performed on a total of 46 CBCT scans selected for this purpose. Scans were split into fully dentate and partially dentate patients (small edentulous regions). The AI Driven tool (Virtual Patient Creator, Relu BV, Leuven, Belgium) automatically detected, segmented and labelled teeth and edentulous regions. Human performance served as clinical reference. Accuracy and speed of the AI-driven tool to detect and label teeth and edentulous regions in partially edentulous jaws were assessed. Automatic tooth segmentation was compared to manually refined segmentation and accuracy by means of Intersetion over Union (IoU) and 95% Hausdorff Distance served as a secondary outcome.
Results
The AI-driven tool achieved a general accuracy of 99.7% and 99% for detection and labelling of teeth and missing teeth for both fully dentate and partially dentate patients, respectively. Automated detections took a median time of 1.5s, while the human operator median time was 98s (P<0.0001). Segmentation accuracy measured by Intersection over Union was 0.96 and 0.97 for fully dentate and partially edentulous jaws respectively.
Conclusions
The AI-driven tool was accurate and fast for CBCT-based detection, segmentation and labelling of teeth and missing teeth in partial edentulism.
Clinical Significance
The use of AI may represent a promising time-saving tool serving radiological reporting, with a major step forward towards automated dental charting, as well as surgical and treatment planning.
Introduction
The speedy improvement in computer technology, the capacity of storing large amounts of information have allowed the gathering of data with greater variety and the formation of big databases, also known as “Big Data”, for dental and medical researches in general [1]. Big Data together with the increasing computer performance have brought Artificial Intelligence (AI) back to the arena. AI is a general term coined to describe the development of computer systems aiming to perform tasks that require human cognition, enhancing the human-machine interaction. New AI techniques, such as Machine Learning (ML), are able to learn through inputs, analyze and extract statistical patterns in complex data and accomplish tasks, such as detection of diseases, image segmentation and enhancement [[2], [3], [4], [5], [6], [7]].
Deep Learning (DL) is a subset of AI that allows computational models composed by multiple processing layers to learn the complex statistical pattern in data with multiple levels of abstraction. DL is structured as a nested hierarchy of concepts in which the underlying composition is made of artificial neural networks (ANN). ANN are layers of processing units known as neurons, sequentially organized via weighted connections. There are plenty of types of deep neural networks. The ones specialised in dealing with grid-like topology data, such as 2D and 3D images, are called Convolutional Neural Networks (CNN) [5,[7], [8], [9]]. Currently, computer-aided diagnosis may assist radiologists to interpret images in order to attain precise and time saving identification and classification of teeth, meanwhile avoiding incorporation of human errors associated with either lack of specialised training, or lack of attention to detailed diagnosis, or fatigue [4,10,11].
AI-based tools have proven to be accurate and fast for detection, segmentation and classification of teeth on 2D imaging, even in the presence of dental treatment such as dental fillings and root canal treatment. It has also been implemented for detection, classification, or disease diagnosis, as well as classification of jaw bone morphology [[12], [13], [14], [15]].
Also some notable AI applications on 3D images found in the literature include the detection, segmentation and classification of the teeth, mandibular canal, pharyngeal airspace and mandibular bone [[16], [17], [18], [19]].
Cone-Beam Computed Tomography (CBCT) has replaced Multi-Slice Computed Tomography (MSCT) due to its major advantages over the latter, such as three-dimensional view of the maxillofacial structures at reasonable dose of radiation, low cost, ease-of-use and more comfortable for patient and operator. Its 3D reconstruction and spatial information provided has been used for planning purposes in many fields of dentistry [[20], [21], [22], [23], [24], [25], [26]].
As a resource for dental assessments, diagnoses and outcomes evaluation, the CBCT scans need to be interpreted by a radiologist who will then elaborate the report. If the computer could identify and label the oral condition correctly prior to the radiologist's reading, it would reduce the time necessary for image interpretations. Accurate detection, segmentation and labelling of teeth and edentulous regions might contribute to further development of computer-assisted and automated dental charting and dental treatment planning. However, scientific reports on computer-assisted detection and labelling of teeth on CBCTs are scarce. If present, these often lack challenging situations, such as in partial edentulism [27,28].
Thus, the aim of this study was to assess the performance and clinically validate a novel AI-driven tool for accurate detection and classification of teeth and small edentulous areas on CBCT. Accuracy of the system to detect and classify teeth/missing teeth were the primary outcomes, time analysis and accuracy of tooth segmentation were secondary outcomes. The hypothesis of the present study was that this novel AI-driven tool would be accurate for detecting and labelling teeth and small edentulous regions with minimal time consumption.
Section snippets
Materials and methods
The present study was designed as an observational study to test accuracy of an AI-based tool for tooth classification and segmentation on CBCTs of fully and partially dentate patients. CBCT data used in this study were retrieved from the Center of Dentomaxillofacial Radiology of the University Hospitals, from March 2016 to January 2021. This study was approved by the Medical Ethical Committee of the UZ Leuven (B322201525552) and was conducted according to the ICH-GCP principles and the
Results
Considering the validation dataset consisting of 46 CBCT scans, 1.472 labels were established as a ground truth by a dental specialist (tooth number or missing tooth).
For fully dentate patients, 736 labels were established. The system was capable of agreeing with the dental specialist on 734 and failed in 2. There were 2 FN. Therefore, the system achieved a general accuracy of 99.7%, 99.7% of recall and 100% of precision.
Also 736 labels were established for patients presenting small edentulous
Discussion
Applications of Artificial Intelligence in dentistry have been investigated considering its potential for fast, accurate and consistent performance of clinical tasks, such as detection, classification and segmentation of orofacial structures and to provide accurate diagnoses. However, currently, the use of AI in clinical dentistry is still limited to specific tasks, such as automatic detection of diseases, image segmentation and imaging resolution enhancement. 3D segmentation of oral structures
Conclusions
The AI-tool presented in this study is accurate and fast for automatic detection and classification of teeth and small edentulous areas on CBCT scans. Its performance is comparable to a human expert, yet more consistent.
Credit authorship contribution statement
Maurício do Nascimento Gerhardt: Methodology, Validation, Formal analysis, Investigation, Data curation, Writing – original draft, Writing – review & editing, Visualization. Rocharles Cavalcante Fontenele: Methodology, Validation, Formal analysis, Investigation, Data curation, Writing – review & editing. André Ferreira Leite: Conceptualization, Methodology, Formal analysis, Investigation, Data curation, Writing – review & editing. Pierre Lahoud: Conceptualization, Methodology, Validation,
Declaration of Competing Interest
The authors declare the following financial interests/personal relationships which may be considered as potential competing interests.
Acknowledgments
This publication was made possible with the support of a Development Project of VLAIO (Flanders Innovation & Entrepreneurship).
References (33)
- F. Schwendicke et al.Convolutional neural networks for dental image diagnostics: a scoping review J. Dent. (2019)
- K. DoiComputer-aided diagnosis in medical imaging: historical review, current status and future potential Comput. Med. Imaging Graph. Off. J. Comput. Med. Imaging Soc. (2007)
- S.B. Khanagar et al.Developments, application, and performance of artificial intelligence in dentistry - a systematic review J. Dent. Sci. (2021)
- P.L. Lin et al.Teeth segmentation of dental periapical radiographs based on local singularity analysis Comput. Methods Programs Biomed. (2014)
- S Vinayahalingam et al.Automated chart filing on panoramic radiographs using deep learning J. Dent. (2021 Dec)
- E Shaheen et al.A novel deep learning system for multi-class tooth segmentation and classification on cone beam computed tomography. A validation study J. Dent. (2021 Dec)
- P Lahoud et al.Development and validation of a novel artificial intelligence driven tool for accurate mandibular canal segmentation on CBCT J. Dent. (2022 Jan)
- S Shujaat et al.Automatic segmentation of the pharyngeal airway space with convolutional neural network J. Dent. (2021 Aug)
- S. Chogle et al.The recommendation of cone-beam computed tomography and its effect on endodontic diagnosis and treatment planning J. Endod. (2020)
- Y. Miki et al.Classification of teeth in cone-beam CT using deep convolutional neural network Comput Biol Med. (2017)
There are more references available in the full-text version of this article.