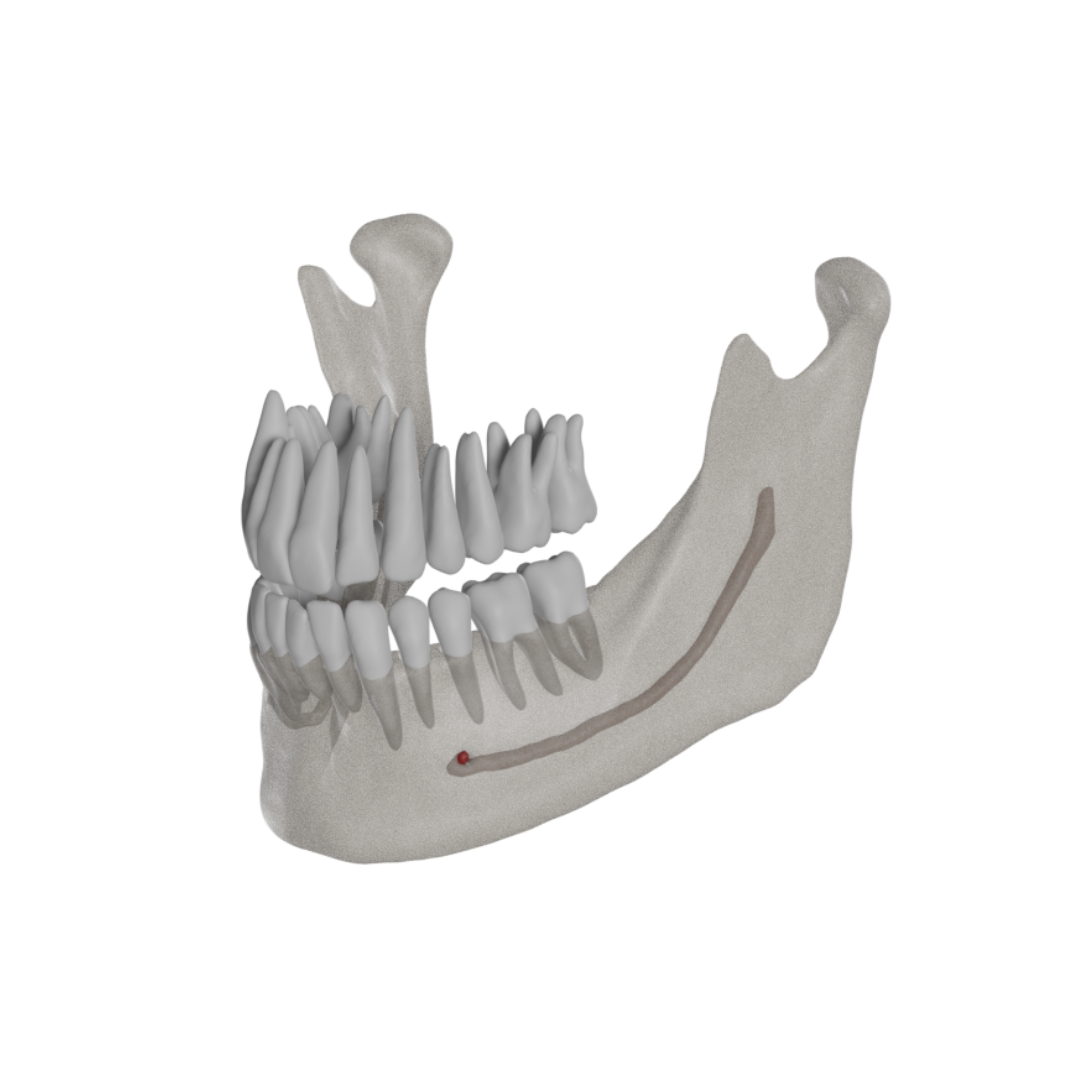
Link to paper
Development and validation of a novel artificial intelligence driven tool for accurate mandibular canal segmentation on CBCT
Pierre Lahoud ab, Siebe Diels c, Liselot Niclaes a, Stijn Van Aelst a, Holger Willems c, Adriaan Van Gerven c, Marc Quirynen b, Reinhilde Jacobs a d
a OMFS-IMPATH Research Group, Department of Imaging and Pathology, Faculty of Medicine, KU Leuven & Department of Oral and Maxillofacial Surgery, University Hospitals Leuven, Belgium
b Department of Oral Health Sciences, Periodontology and Oral Microbiology, University Hospitals of Leuven, Belgium
c Relu BV, Leuven, Belgium
d Department of Dental Medicine, Karolinska Institute, Stockholm, Sweden
Abstract
Objectives
The objective of this study is the development and validation of a novel artificial intelligence driven tool for fast and accurate mandibular canal segmentation on cone beam computed tomography (CBCT).
Methods
A total of 235 CBCT scans from dentate subjects needing oral surgery were used in this study, allowing for development, training and validation of a deep learning algorithm for automated mandibular canal (MC) segmentation on CBCT. Shape, diameter and direction of the MC were adjusted on all CBCT slices using a voxel-wise approach. Validation was then performed on a random set of 30 CBCTs - previously unseen by the algorithm - where voxel-level annotations allowed for assessment of all MC segmentations.
Results
Primary results show successful implementation of the AI algorithm for segmentation of the MC with a mean IoU of 0.636 (± 0.081), a median IoU of 0.639 (± 0.081), a mean Dice Similarity Coefficient of 0.774 (± 0.062). Precision, recall and accuracy had mean values of 0.782 (± 0.121), 0.792 (± 0.108) and 0.99 (± 7.64×10−05) respectively. The total time for automated AI segmentation was 21.26 s (±2.79), which is 107 times faster than accurate manual segmentation.
Conclusions
This study demonstrates a novel, fast and accurate AI-driven module for MC segmentation on CBCT.
Clinical Significance
Given the importance of adequate pre-operative mandibular canal assessment, Artificial Intelligence could help relieve practitioners from the delicate and time-consuming task of manually tracing and segmenting this structure, helping prevent per- and post-operative neurovascular complications.
Introduction
The last two decades have seen a shift towards full digital workflows for pretreatment diagnostics, treatment planning and follow-up [1,2]. In this regard, Cone Beam Computed Tomography (CBCT) has gained a prominent position in this workflow, considering the low costs and compact size of such machines, meanwhile providing essential 3D anatomical details with high spatial resolution and low radiation dose [2,3]. CBCT allows visualization of critical anatomical structures, such as the mandibular canal (MC), housing the vital mandibular neurovascular bundle [4,5]. Knowledge of the exact position of the MC and its relation to adjacent structures is crucial to help avoiding mild to severe life-altering conditions [4], [5], [6] during implant placement [4,5], sagittal split osteotomy [4,7], cyst removal [4,8] and tooth extraction [4,5,7,9]. Such injuries are relatively common (incidence from 0.2 to 8.4%) [9,10], and could lead to (semi)-permanent paresthesia, anesthesia or dysesthesia of the innervated structures of the affected side (such as lip, jaw, teeth, tongue, mucosa, gingiva) [4,8]. Iatrogenic trigeminal damage could also significantly impact quality of life, lifestyle and psychosocial outcomes [11].
Accurate pre-operative assessment of the MC and any potential anatomical variation of this structure is thus crucial to avoid post-operative complications and damage to the inferior alveolar nerve [4,7]. Yet, precise and automatic delineation of the MC remains challenging. Several CBCT-guided planning software tools allow for visualization of the MC after manual placement of marks at different locations across the canal's path with interpolation into a fixed diameter cylinder, providing a virtual depiction the MC, denoted as MC tracing.
Nevertheless, this approach yields certain inherent inaccuracies given the fully manual nature of the tracing [12,13], coupled with the inherent limitations of CBCTs in terms of low image contrast, increased noise, artefacts and the lack of Hounsfield units. All of this adds to the complexity of achieving - manually or automatically – an accurate assessment of this structure [3,14].
For these reasons, the introduction of Convolutional Neural Networks (CNNs) and Artificial Intelligence (AI) in medical imaging segmentation tasks has been seen as an apparent solution for countering such problems: CNNs, which are at the core of AI technologies, are computational processing systems heavily inspired by how the occipital cortex operates. They are comprised of neurons that self-optimize through learning, consisting of an input and an output layer, with multiple hidden layers in between. They are primarily used in the field of patterns recognition within images [15].
CNNs and AI have proven to counter various limitations previously met with manual and automatic segmentation methods [16], [17], [18], which could in our case allow for accurate automated segmentation of the MC, in spite of localized morphological variations such as bifid canals and localized canal enlargement, assisting clinicians in accurate presurgical MC assessment; thus lowering the risk of per-and postoperative complications.
Therefore, the aim of this study is twofold: 1. development and validation of a novel tool for accurate voxel-wise segmentations, capable of adjusting to variations in MC shape and width MC; 2. training and automation of such a tool for fast and accurate result generation. The hypothesis is that such AI-driven tool might provide MC segmentations for clinical use, that are much faster and at least as accurate as the expert's segmentations.
Section snippets
Data acquisition and training database
A random collection of CBCT scans from the M3BE database (Ethical Committee Research UZ/KU Leuven B322201525552) was initially gathered, yielding a total of 235 CBCT scans from dentate patients needing oral surgical procedures (mean age 25±11 years old). Scans were acquired using the 3D Accuitomo 170 (Morita, Kyoto, Japan), ProMax 3D MAX (Planmeca, Helsinki, Finland), Scanora 3Dx (Soredex, Tuusula, Finland) and NewTom VGI EVO (QR Verona, Cefla, Verona, Italy) devices (Table 1). An initial
Results
Results of IoU, DSC, Precision, Recall, Accuracy and HD can be found in Table 2. The mean IoU of the 30 cases was 0.636 (± 0.081), the median IoU 0.639 (± 0.081), the mean DSC was 0.782 (±0.062). HD had a mean value of 0.705 mm (±0.389), while Precision, Recall and Accuracy had mean values of 0.782 (±0.121), 0.792 (±0.108) and 0.99 (±9.52 × 10−05) respectively Total time from uploading until result visualization was 21.26 s (±2.79), while the average time for expert manual segmentation was
Discussion
Accurate MC assessment for procedures similar to tooth extraction, implant placement, bone grafting and orthognathic surgeries have proven to be of high clinical relevance [13,20,30]. Assessing structures using imaging modalities, such as CBCTs can be done through segmentation.
However, limitations of conventional segmentation techniques for surgical assessment in both the medical and dental fields have led in the last few years to a surge in DL technologies for classification and segmentation
Conclusion
The present study introduced development and validation of a novel AI-driven tool for fast and accurate mandibular canal segmentation on CBCT. The results obtained in this study could help improving pre-surgical planning procedures, such as for implant placement, bone grafting, orthognathic surgery and tooth extraction. The developed technique may open further doors for advanced AI development to automatically visualize accessory canals, anatomical variations as well as neurovascularisation in
Credit authorship contribution statement
P. Lahoud: Conceptualization, Methodology, Validation, Formal Analysis, Investigation, Interpretation, Writing - Original Draft, Writing - Review & Editing.
S. Diels: Conceptualization, Methodology, Validation, Software, Writing - Original Draft, Writing - Review & Editing.
L. Niclaes: Formal Analysis, Investigation, Interpretation, Writing - Review & Editing.
S. Van Aelst: Formal Analysis, Investigation, Interpretation, Writing - Review & Editing.
H. Willems: Conceptualization, Methodology,
Declaration of Competing Interest
The authors declare that they have no known competing financial interests or personal relationships that could have appeared to influence the work reported in this paper.
References (35)
- J.B. Carter et al. Applications of cone-beam computed tomography in oral and maxillofacial surgery: an overview of published indications and clinical usage in United States academic centers and oral and maxillofacial surgery practices J. Oral. Maxillofac Surg. (2016)
- H. Ghaeminia et al .The use of cone beam CT for the removal of wisdom teeth changes the surgical approach compared with panoramic radiography: a pilot study Int. J. Oral Maxillofac Surg. (2011)
- B. Friedland et al. The use of 3-dimensional reconstructions to evaluate the anatomic relationship of the mandibular canal and impacted mandibular third molars J. Oral Maxillofac Surg. (2008)
- Y.Y. Leung et al. Risk factors of neurosensory deficits in lower third molar surgery: an literature review of prospective studies Int. J. Oral Maxillofac Surg. (2011)
- M. Ueda et al. Clinical significance of computed tomographic assessment and anatomic features of the inferior alveolar canal as risk factors for injury of the inferior alveolar nerve at third molar surgery J. Oral Maxillofac Surg. (2012)
- M.A. Pogrel et al. Lingual flap retraction for third molar removal J. Oral Maxillofac Surg. (2004)
- N.L. Gerlach et al. Reproducibility of 3 different tracing methods based on cone beam computed tomography in determining the anatomical position of the mandibular canal J. Oral Maxillofac Surg. (2010)
- N.L. Gerlach et al. Accuracy of assessing the mandibular canal on cone-beam computed tomography: a validation study J. Oral Maxillofac. Surg. (2014)
- I. Balki et al. Sample-size determination methodologies for machine learning in medical imaging research: a systematic review Can. Assoc. Radiol. J. (2019)
- Y. Tsuji et al. Computed tomographic analysis of the position and course of the mandibular canal: relevance to the sagittal split ramus osteotomy Int. J. Oral Maxillofac. Surg. (2005)